Modelling
Models are simplified descriptions of the real world. They depict the functioning of ecosystems and complement individual observations. Comparing model results to observations provides insights into the reliability of the model.
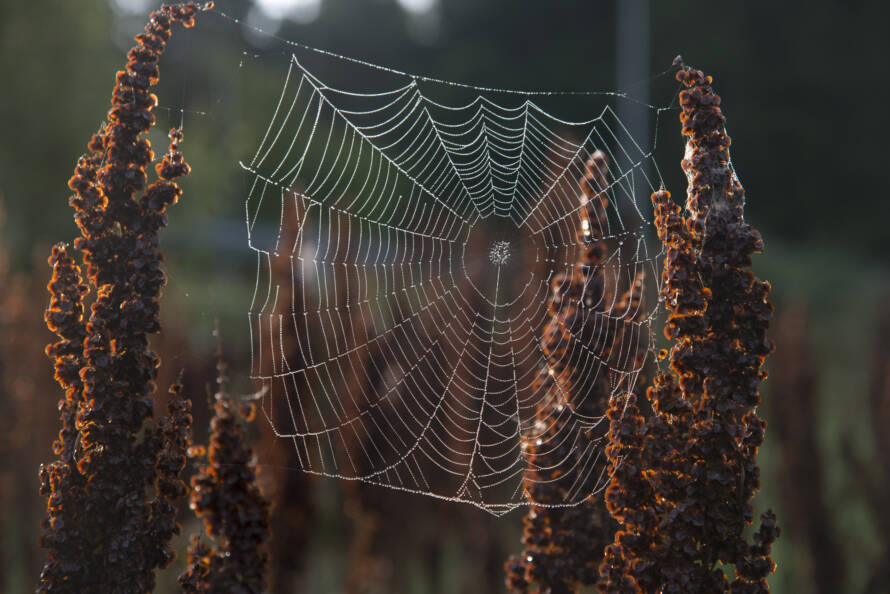
On this page:
- Modeling provides information on changes in the state of nature and supports conservation planning
- What are the models used for?
- A wide range of modeling methods
- Statistical models and machine learning models
- Dynamic species population models and process-based ecosystem models
- Modelling uncertainty
- A model is a simplified description of reality
- Sources
Modeling provides information on changes in the state of nature and supports conservation planning
Various models describing the state of nature and diversity produce information for planning the protection and management of species and habitats, as well as supporting decision-making. The models describe the functioning of habitats and supplement individual observations. Models also help to show variations of species populations, habitat types and the state of nature in time and space.
Models can be used to monitor and predict the effects of land use, climate change and other human-caused environmental changes on diversity and the functioning of ecosystems. Information about the functionality and reliability of the models is obtained by comparing the results given by the models with the observations. The uncertainty of the models can be reduced by collecting more data in the field and using that to develop a better model.
What are the models used for?
The models can be used to produce both basic and applied information about the functioning, structure and internal cause-and-effect chains of ecosystems and species communities, as well as the environmental factors that control the development, spread and survival of species individuals and populations. Human activity often has a significant impact on biodiversity. This effect can be visualized with the help of models; the predictions of the models can be used as an aid in social planning and decision-making.
The regional assessments of the nature effects of land use and the allocation of protection measures to nature areas that are significant in terms of species and habitat types or sensitive to adverse effects are based on the available information. Since not all areas can be mapped and not all characteristics of habitats can be measured, models are used as support. Models help to fill the gaps in nature and ecosystem knowledge, to identify connections between different factors and to assess the direction and intensity of future changes. Models are based on existing knowledge, understanding and measurements of the subject under consideration.
Models can be used to study for example the connections between ecosystems, species communities and climate and related processes. They can be used to predict future changes in various natural features for example how the changing environment and land use decisions made now will affect biodiversity. Modeling provides one additional means of monitoring ecosystems and species populations.
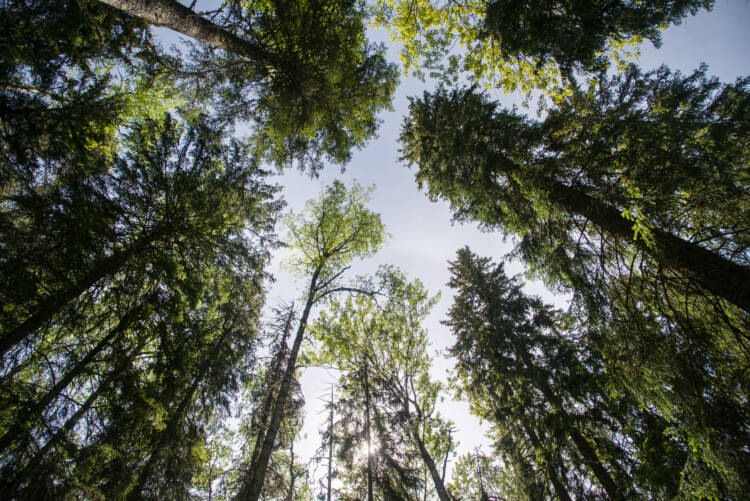
A wide range of modeling methods
There is a wide range of approaches and techniques used in modeling species, habitats, and other aspects of biodiversity. Modeling methods can be classified in different ways; one classification is to divide them into statistical and process-based models. The wide spectrum of models is also indicated by the fact that in one of the most recent comparative studies (Nordberg et al. 2019) more than 30 modeling methods of species occurrence areas based on statistical, or machine learning methods have been included.
Statistical models and machine learning models
There are dozens of uses of statistical models in ecology and conservation biology. The models help, for example, to understand how the occurrence of valuable natural features depends on environmental factors and how different land use methods and other human measures that shape nature affect them. Compilation of this type of model is based on information on where the natural feature in question occurs and where it does not, as well as information about environmental variables measured (or otherwise assessable) from these places.
Statistical models are ‘static’, mathematical equations describing correlations between variables. They tell about how the occurrence or variation of a phenomenon or variable is linked to the variation of other biotic or abiotic factors.
In the models, the environmental variables act as factors explaining the variation in the response variable, such as the presence or abundance of rare species or other natural features. A wide variety of variables can be used as explanatory environmental factors, such as variables describing the macroclimate or the local climate, the structure, coverage and quality characteristics of the vegetation of the place of occurrence, the moisture and nutrient properties of the soil or the land use of the surrounding landscape (e.g. Ilmonen et al. 2009; Pecchi et al. 2019; Saarimaa et al. 2019 ; Virkkala et al. 2022).
It should be noted that statistical models based on regression analyzes tell about the correlations between the response variable and the explanatory factors, and they do not necessarily describe cause-effect-type causality relationships (Jarnevich et al. 2015). This caution is also essential for models other than regression models. Models should be evaluated carefully, especially in the study of poorly known species and also when the available environmental variables have been measured approximately or they are only indirectly or distally connected to ecological variables central to the species (so-called ‘distal’ or ‘surrogate predictors’; Hof et al. 2012; Mod et al. . 2016; Gardner et al. 2019).
Often, previous research or a general understanding of the ecological foundations of species and habitat types provides a sufficient basis for statistical modeling of natural features. With models based on ecologically important environmental variables, it is thus possible to predict places favorable for the occurrence of conservationally significant natural features in poorly known areas, as well as how changes in climate and land use affect their occurrence.
One significant use of statistical models and the data they produce in Finland has been to predict the locations of sites valuable in terms of forest and wetland conservation (e.g. Parviainen et al. 2008; Saarimaa et al. 2019; Björklund et al. 2020; Forsius et al. 2021; Virkkala et al. 2022; Kujala et al. 2023). Statistical diversity models can also be used to target field surveys to the most promising new locations (Roden et al. 2017; Rosner-Katz et al. 2020).
Models used in ecological research
Linear regression models
The most traditional models used in ecological research are linear regression models (Zuur et al. 2007). They can be used to assess for example whether the individual density of a single species or species changes as a result of different climate variables or environmental variables, either linearly increasing or decreasing, or whether the change is minor and not statistically significant. Often only observed/not observed level information is available on species occurrence. One traditional method for modeling such data is logistic regression.
General modeling methods (GLM and GAM)
In recent decades, certain flexible, general modeling methods have gained a strong position in the modeling of species occurrence and abundance variation; these are the so-called generalized linear models (GLM; McCullagh 2019) and generalized additive models (GAM; Hastie & Tibshirani 1990). Both GLM and GAM are combinations of modeling algorithms that can be used to model different types of response variables based on different statistical distributions. With the help of these methods, the user can also flexibly change the assumed form of the influence of explanatory environmental factors to the modeled response variable, i.e. the so-called response ratio. Instead of a linear relationship, non-linear, curvilinear, or even meandering response assumptions can be used in different ways; In GLM, this happens with the help of polynomial terms of the variables, and in GAM models with so-called smooth functions (Guisan et al. 2002; Clark & Wells 2023).
Machine learning models
The so-called machine learning models (Crisci et al. 2012) are also very flexible methods regarding the assumptions of the relationship between the response variable and explanatory environmental factors. These models work iteratively, whereby the accuracy of the model is continuously improved. In machine learning models based on the classification of modeling data, hundreds of different classification trees can be made that explain the variation of the response variable, branching into smaller and smaller features. Based on the accuracy of these classification trees, machine learning programs improve the structure of the model through an iterative process until the best possible classification or prediction accuracy is achieved.
Machine learning methods often used in the modeling of biodiversity data include, among others
- Random Forest (Cutler et al. 2007; Heikkinen et al. 2010)
- Boosted regression trees (BRT; Elith et al. 2008)
At best, these programs are able to produce quite detailed and functional forecasts of the presence of places favorable to species or of a resource central to biodiversity, such as important habitats, decaying wood, individuals of large-sized key tree species or microclimates with special conditions.
Dynamic species population models and process-based ecosystem models
Dynamic species population models
Species-level process models are typically models describing different processes of species populations, such as demographic changes, variation in the viability of individuals, and distribution. Step-by-step dynamic species models can be used to assess the impact of various environmental factors and species life-history traits on the demographic processes of species populations and the spread of species (Bocedi et al. 2014; Heikkinen et al. 2014, 2015).
Dynamic species models can be applied both to artificial, simulated data and to field study data collected from the research area. In the latter case, the simulation predictions produced by the dynamic models describe
- how well the individuals of the species are able to move to new places within the study area
- under what conditions new permanent local populations can arise
- how well the current occurrences are preserved in changing conditions
- how variations in conditions between years affect the viability of populations
Carefully parameterized dynamic species models also help assessing how different types of species will succeed under recent and projected future climate and land-use changes, as well as how habitat management efforts can support the maintenance of regional populations.
Process-based ecosystem models
Process-based ecosystem models can be used to describe, for example, the effects of environmental factors and human activity on the ecosystem being studied. Process-based ecosystem models describe the structure of the system under consideration, the cause-and-effect relationships between its parts and changes in the system over time as mathematical functions.
Process-based ecosystem models can be used to generate information about variables for which there are only few direct measurements, but the influencing factors are sufficiently well known, or when you want to make predictions. For example, it is difficult to obtain sufficiently comprehensive measurement data on the amount of decaying wood in forests. However, with a process model that describes the growth and mortality of trees, it is possible to estimate the accumulation of rotten wood based on detailed and comprehensive information about the structural characteristics of forests. Modelled estimates of structural features of living and dead trees can also be used, for example, in modeling bird populations.
The process-based PREBAS model, which describes the growth and carbon balance of forests, was used to study the effect of logging volumes on the development of carbon sinks and stores in forests as a collaboration of the FEO, IBC-Carbon and SysteemiHiili projects.
The PREBAS model developed at the University of Helsinki is designed to describe and predict forest carbon cycle and tree growth especially in Finland, but also more generally in boreal forests. With the PREBAS model, it is possible to evaluate the change in forest vegetation and soil carbon stock:
- growth
- photosynthesis
- gas exchange
- carbon storage and loss
The calculation based on the location of the forest and the development of the vegetation gives a map-based estimate of the forest’s carbon sink and carbon storage. The PREBAS model is suitable for assessing the effects of climate change and forest management measures in large areas, such as at the level of a province or the entire country. The starting data of the model is forest survey data and the so-called driving variables are weather data.
Modelling uncertainty
Since both GLM and GAM models and machine learning methods can produce quite complicated, possibly overparameterized biodiversity models, their use requires caution. First, if the material available for modeling contains parts or variables of uncertain quality, uncertain predictions can be produced. Second, over-parameterized models or models with uncertain response ratios often work accurately within the ‘ecological state’ of the model’s calibration area, but at the edges and especially outside of it, the reliability of the predictions produced by the model may decrease (Heikkinen et al. 2012; Yates et al. 2018).
When predicting favorable locations for species in unmapped areas (Virkkala et al. 2022) and in various land use situations (Luoto et al. 2007; Seedre et al. 2018) and in a changing climate (Virkkala et al. 2008, 2014; Eskildsen et al. 2013), there are other potential uncertainties in the use of models. These include, among others
- strong correlation between environmental variables
- spatial autocorrelation structure of species occurrences and environmental variables
- interaction effects between variables
- limited applicability of model algorithms to different research data (Jarnevich et al. 2015; Bryn et al. 2021).
Ways to manage uncertainties
There are several ways to manage model uncertainties, such as the following three:
- careful evaluation of the ecological logic of the response variable and explanatory factors of the models
- cross-testing of model forecasts, i.e. cross-validation, so that the accuracy of the forecasts is assessed with data that has not been used in model calibration
- by modeling with several different methods and/or different combinations of variables and then combining the predictions of the models (the so-called ensemble model; Marmion et al. 2009; Hällfors et al. 2016). This brings out the areas for which the forecasts of several models are consistent and therefore more reliable than on average.
In statistical models of species occurrence areas it is important to consider whether the species data includes records of both the presence of the species and the fact that it has not been observed, or whether it consists only of ‘positive’ observations (so-called ‘presence/absence models’ vs. ‘presence-only models’; Elith et al. 2020). Observation data collected by natural history museums and data based on citizen science often contain only positive species observations. The method often used in the modeling of this type of data is Maxent, which was developed specifically to take into account the special features of ‘presence-only’ data (Merow et al. 2013). In the application of other modeling methods, the lack of occurrence data can be taken into account by various means such as generating artificial species-not-observed data (‘pseudoabsences’; Barbet-Massin et al. 2012) in the modeling data according to careful consideration.
Modeling occurrences of rare species often presents special challenges. In their modeling, treating the species as a single community to be modeled can be a significant help. These types of models are known as joint species distribution models, where the method combines different species into interdependent modeling response variables.
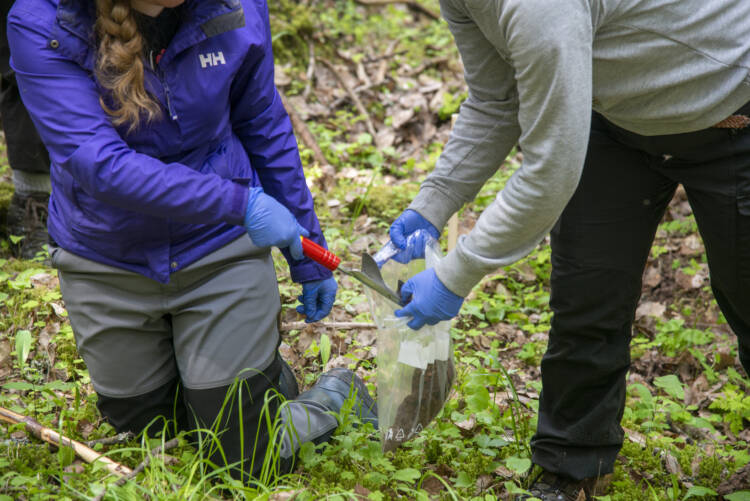
A model is a simplified description of reality
Models are simplified descriptions of the real world. By comparing the results of the model with the observations, we get information about the reliability of the model. The uncertainty of models can be reduced with the help of measurement data and model development.
Uncertainty is part of natural scientific measurements and modeling, which must be taken into account when interpreting the results of models and in decision-making based on modelling. Uncertainty means in particular that the magnitude of the investigated variable is not known precisely but only with a range or probability, but also that the processes and variables related to the investigated phenomenon have not been identified well enough.
If the uncertainty of models is not recognized, wrong assumptions can be made about the consequences of decisions based on modeling. When the uncertainties of the models are carefully taken into account, a basis is created to identify the measures that are most likely to lead to the goals set for example for conservation planning and nature management.
The uncertainty of the input data or the initial state of models that examine the functioning of ecosystems can be evaluated with the help of measurements. Since measurement data is usually only available from some places and at some moments, the measurement data must be generalized to improve the spatial and temporal coverage of the data. The measurements can also be used to calibrate models and determine their structural uncertainty.
However, uncertainty can be caused by things that are not known or cannot be measured, such as future development. In such cases, we can use scenario analyses, which allow us to outline and compare alternative development paths depending on different assumptions. By simulating the development of forests several times in a row with randomly varying initial data, we can also identify solutions that always lead to the same final result, regardless of the uncertainty of the model and its initial data. For example, we can identify areas whose nature values are most likely to be preserved in the future, regardless of modeling uncertainty.
The inclusion of uncertainties in the research results indicates the quality and usability of the research as a support for decision-making. In addition, it helps to understand the limitations of the research. It is not always possible to reduce the uncertainty associated with model estimates. Then it is important to consider how big and what kind of risk we are ready to take. In this case, applying the precautionary principle and choosing the least risky option may make the most sense.
Sources
Barbet-Massin, M., Jiguet, F., Albert, C.H., Thuiller, W., 2012. Selecting pseudo-absences for species distribution models: how, where and how many? Methods in Ecology and Evolution 3, 327-338.
Bocedi, G., Palmer, S.C.F., Pe’er, G., Heikkinen, R.K., Matsinos, Y.G., Watts, K., Travis, J.M.J., 2014. RangeShifter: a platform for modelling spatial eco-evolutionary dynamics and species’ responses to environmental changes. Methods in Ecology and Evolution 5, 388-396.
Bryn, A., Bekkby, T., Rinde, E., Gundersen, H., Halvorsen, R., 2021. Reliability in Distribution Modeling—A Synthesis and Step-by-Step Guidelines for Improved Practice. Frontiers in Ecology and Evolution 9.
Clark, N.J., Wells, K., 2023. Dynamic generalised additive models (DGAMs) for forecasting discrete ecological time series. Methods in Ecology and Evolution 14, 771-784.
Crisci, C., Ghattas, B., Perera, G., 2012. A review of supervised machine learning algorithms and their applications to ecological data. Ecological Modelling 240, 113-122.
Cutler, D.R., Edwards Jr., T.C., Beard, K.H., Cutler, A., Hess, K.T., Gibson, J., Lawler, J.J., 2007. Random forests for classification in ecology. Ecology 88, 2783-2792.
Elith, J., Leathwick, J.R., Hastie, T., 2008. A working guide to boosted regression trees. Journal of Animal Ecology 77, 802-813.
Elith, J., Graham, C., Valavi, R., Abegg, M., Bruce, C., Ferrier, S., Ford, A., Guisan, A., Hijmans, R.J., Huettmann, F., Lohmann, L., Loiselle, B., Moritz, C., Overton, J., Peterson, A.T., Phillips, S., Richardson, K., Williams, S., Wiser, S.K., Wohlgemuth, T., Zimmermann, N.E., 2020. Presence-only and Presence-absence Data for Comparing Species Distribution Modeling Methods. Biodiversity Informatics 15, 69-80.
Eskildsen, A., le Roux, P.C., Heikkinen, R.K., Høye, T.T., Kissling, W.D., Pöyry, J., Wisz, M.S., Luoto, M., 2013. Testing species distribution models across space and time: high latitude butterflies and recent warming. Global Ecology and Biogeography 22, 1293-1303.
Gardner, A.S., Maclean, I.M.D., Gaston, K.J., 2019. Climatic predictors of species distributions neglect biophysiologically meaningful variables. Diversity and Distributions 25, 1318-1333.
Guisan, A., Edwards, T.C.J., Hastie, T., 2002. Generalized linear and generalized additive models in studies of species distributions: setting the scene. Ecological Modelling 157, 89-100.
Hastie, T., Tibshirani, R., 1990. Generalized additive models. Chapman and Hall, London. 335 pp.
Heikkinen, R.K., Luoto, M., Leikola, N., Pöyry, J., Settele, J., Kudrna, O., Marmion, M., Fronzek, S., Thuiller, W., 2010. Assessing the vulnerability of European butterflies to climate change using multiple criteria. Biodiversity and Conservation 19, 695-723.
Heikkinen, R.K., Marmion, M., Luoto, M., 2012. Does the interpolation accuracy of species distribution models come at the expense of transferability? Ecography 35, 276-288.
Heikkinen, R.K., Bocedi, G., Kuussaari, M., Heliölä, J., Leikola, N., Pöyry, J., Travis, J.M.J., 2014. Impacts of Land Cover Data Selection and Trait Parameterisation on Dynamic Modelling of Species’ Range Expansion. Plos One 9, e108436.
Heikkinen, R.K., Pöyry, J., Virkkala, R., Bocedi, G., Kuussaari, M., Schweiger, O., Settele, J., Travis, J.M.J., 2015. Modelling potential success of conservation translocations of a specialist grassland butterfly. Biological Conservation 192, 200-206.
Hof, A.R., Jansson, R., Nilsson, C., 2012. The usefulness of elevation as a predictor variable in species distribution modelling. Ecological Modelling 246, 86-90.
Ilmonen, J., Paasivirta, L., Virtanen, R., Muotka, T., 2009. Regional and local drivers of macroinvertebrate assemblages in boreal springs. Journal of Biogeography 36, 822-834.
Jarnevich, C.S., Stohlgren, T.J., Kumar, S., Morisette, J.T., Holcombe, T.R., 2015. Caveats for correlative species distribution modeling. Ecological Informatics 29, 6-15.
Luoto, M., Virkkala, R., Heikkinen, R.K., 2007. The role of land cover in bioclimatic models depends on spatial resolution. Global Ecology and Biogeography 16, 34-42.
Marmion, M., Parviainen, M., Luoto, M., Heikkinen, R.K., Thuiller, W., 2009. Evaluation of consensus methods in predictive species distribution modelling. Diversity and Distributions 15, 59-69.
McCullagh, P., 2019. Generalized linear models. Routledge, New York. 532 pp.
Merow, C., Smith, M.J., Silander Jr, J.A., 2013. A practical guide to MaxEnt for modeling species’ distributions: what it does, and why inputs and settings matter. Ecography 36, 1058-1069.
Mod, H.K., Scherrer, D., Luoto, M., Guisan, A., 2016. What we use is not what we know: environmental predictors in plant distribution models. Journal of Vegetation Science 27, 1308-1322.
Norberg, A., Abrego, N., Blanchet, F.G., Adler, F.R., Anderson, B.J., Anttila, J., Araújo, M.B., Dallas, T., Dunson, D., Elith, J., Foster, S.D., Fox, R., Franklin, J., Godsoe, W., Guisan, A., O’Hara, B., Hill, N.A., Holt, R.D., Hui, F.K.C., Husby, M., Kålås, J.A., Lehikoinen, A., Luoto, M., Mod, H.K., Newell, G., Renner, I., Roslin, T., Soininen, J., Thuiller, W., Vanhatalo, J., Warton, D., White, M., Zimmermann, N.E., Gravel, D., Ovaskainen, O., 2019. A comprehensive evaluation of predictive performance of 33 species distribution models at species and community levels. Ecological Monographs 89, e01370.
Pecchi, M., Marchi, M., Burton, V., Giannetti, F., Moriondo, M., Bernetti, I., Bindi, M., Chirici, G., 2019. Species distribution modelling to support forest management. A literature review. Ecological Modelling 411, 108817.
Rhoden CM, Peterman WE, Taylor CA. 2017. Maxent-directed field surveys identify new populations of narrowly endemic habitat specialists. PeerJ 5:e3632. https://doi.org/10.7717/peerj.3632(you are switching to another service).
Rosner-Katz, H., McCune, J.L., Bennett, J.R., 2020. Using stacked SDMs with accuracy and rarity weighting to optimize surveys for rare plant species. Biodiversity and Conservation 29, 3209-3225.
Saarimaa, M., Aapala, K., Tuominen, S., Karhu, J., Parkkari, M., Tolvanen, A., 2019. Predicting hotspots for threatened plant species in boreal peatlands. Biodiversity and Conservation 28, 1173-1204.
Seedre, M., Felton, A., Lindbladh, M., 2018. What is the impact of continuous cover forestry compared to clearcut forestry on stand-level biodiversity in boreal and temperate forests? A systematic review protocol. Environmental Evidence 7, 28.
Virkkala, R., Heikkinen, R.K., Leikola, N., Luoto, M., 2008. Projected large-scale range reductions of northern-boreal land bird species due to climate change. Biological Conservation 141, 1343-1353.
Virkkala, R., Leikola, N., Kujala, H., Kivinen, S., Hurskainen, P., Kuusela, S., Valkama, J., Heikkinen, R.K., 2022. Developing fine-grained nationwide predictions of valuable forests using biodiversity indicator bird species. Ecological Applications 32, e2505.
Zuur, A. F., Ieno, E. N., & Smith, G. M. (2007). Linear regression. In: Analysing Ecological Data. Statistics for Biology and Health. Springer, New York, NY. https://doi.org/10.1007/978-0-387-45972-1_5(you are switching to another service)
Yates, K.L., Bouchet, P.J., Caley, M.J., Mengersen, K., Randin, C.F., Parnell, S., Fielding, A.H., Bamford, A.J., Ban, S., Barbosa, A., Dormann, C.F., Elith, J., Embling, C.B., Ervin, G.N., Fisher, R., Gould, S., Graf, R.F., Gregr, E.J., Halpin, P.N., Heikkinen, R.K., Heinanen, S., Jones, A.R., Krishnakumar, P.K., Lauria, V., Lozano-Montes, H., Mannocci, L., Mellin, C., Mesgaran, M.B., Moreno-Amat, E., Mormede, S., Novaczek, E., Oppel, S., Crespo, G.O., Peterson, A.T., Rapacciuolo, G., Roberts, J.J., Ross, R.E., Scales, K.L., Schoeman, D., Snelgrove, P., Sundblad, G., Thuiller, W., Torres, L.G., Verbruggen, H., Wang, L., Wenger, S., Whittingham, M.J., Zharikov, Y., Zurell, D., Sequeira, A.M.M., 2018. Outstanding Challenges in the Transferability of Ecological Models. Trends in Ecology & Evolution 33, 790-802.